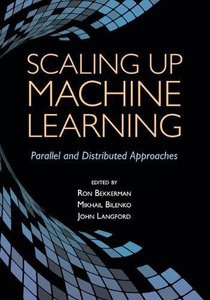
Scaling up Machine Learning: Parallel and Distributed Approaches (Paperback)
內容描述
This book presents an integrated collection of representative approaches for scaling up machine learning and data mining methods on parallel and distributed computing platforms. Demand for parallelizing learning algorithms is highly task-specific: in some settings it is driven by the enormous dataset sizes, in others by model complexity or by real-time performance requirements. Making task-appropriate algorithm and platform choices for large-scale machine learning requires understanding the benefits, trade-offs, and constraints of the available options. Solutions presented in the book cover a range of parallelization platforms from FPGAs and GPUs to multi-core systems and commodity clusters, concurrent programming frameworks including CUDA, MPI, MapReduce, and DryadLINQ, and learning settings (supervised, unsupervised, semi-supervised, and online learning). Extensive coverage of parallelization of boosted trees, SVMs, spectral clustering, belief propagation and other popular learning algorithms and deep dives into several applications make the book equally useful for researchers, students, and practitioners.