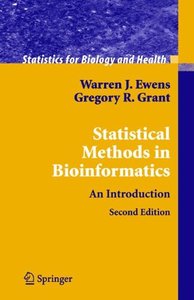
Statistical Methods in Bioinformatics: An Introduction, 2/e
內容描述
Description
Advances in computers and biotechnology have had
a profound impact on biomedical research, and as a result complex data sets
can now be generated to address extremely complex biological questions.
Correspondingly, advances in the statistical methods necessary to analyze such
data are following closely behind the advances in data generation methods. The
statistical methods required by bioinformatics present many new and difficult
problems for the research community.
This book provides an introduction to some of
these new methods. The main biological topics treated include sequence
analysis, BLAST, microarray analysis, gene finding, and the analysis of
evolutionary processes. The main statistical techniques covered include
hypothesis testing and estimation, Poisson processes, Markov models and Hidden
Markov models, and multiple testing methods.
The second edition features new chapters on
microarray analysis and on statistical inference, including a discussion of
ANOVA, and discussions of the statistical theory of motifs and methods based
on the hypergeometric distribution. Much material has been clarified and
reorganized.
The book is written so as to appeal to biologists
and computer scientists who wish to know more about the statistical methods of
the field, as well as to trained statisticians who wish to become involved
with bioinformatics. The earlier chapters introduce the concepts of
probability and statistics at an elementary level, but with an emphasis on
material relevant to later chapters and often not covered in standard
introductory texts. Later chapters should be immediately accessible to the
trained statistician. Sufficient mathematical background consists of
introductory courses in calculus and linear algebra. The basic biological
concepts that are used are explained, or can be understood from the context,
and standard mathematical concepts are summarized in an Appendix. Problems are
provided at the end of each chapter allowing the reader to develop aspects of
the theory outlined in the main text.
Warren J. Ewens holds the Christopher H. Brown
Distinguished Professorship at the University of Pennsylvania. He is the
author of two books, Population Genetics and Mathematical Population Genetics.
He is a senior editor of Annals of Human Genetics and has served on the
editorial boards of Theoretical Population Biology, GENETICS, Proceedings of
the Royal Society B and SIAM Journal in Mathematical Biology. He is a fellow
of the Royal Society and the Australian Academy of Science.
Gregory R. Grant is a senior bioinformatics
researcher in the University of Pennsylvania Computational Biology and
Informatics Laboratory. He obtained his Ph.D. in number theory from the
University of Maryland in 1995 and his Masters in Computer Science from the
University of Pennsylvania in 1999.
Comments on the First Edition. "This book would
be an ideal text for a postgraduate course…[and] is equally well suited to
individual study…. I would recommend the book highly" (Biometrics). "Ewens and
Grant have given us a very welcome introduction to what is behind those pretty
[graphical user] interfaces" (Naturwissenschaften.). "The authors do an
excellent job of presenting the essence of the material without getting bogged
down in mathematical details" (Journal. American Staistical. Association).
"The authors have restructured classical material to a great extent and the
new organization of the different topics is one of the outstanding services of
the book" (Metrika).
Table of
Contents
An Introduction to Probability Theory: One Random Variable An
Introduction to Probability Theory: Many Random Variables Statistics: An
Introduction to Statistical Inference Stochastic Processes: An Introduction
to Poisson Processes and Markov Chains The Analysis of DNA Sequence
Patterns: One sequence The Analysis of DNA Sequences: Multiple sequences
Stochastic Processes: Random Walks Statistics: Classical Estimation and
Hypothesis Testing BLAST Stochastic Processes: Markov Chains Hidden
Markov Models Computationally intensive methods Evolutionary models *
Phylogenetica tree estimation