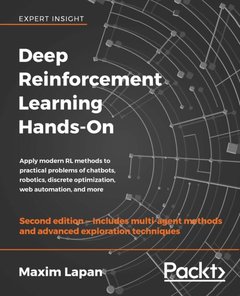
Deep Reinforcement Learning Hands-On - Second Edition
內容描述
Deep Reinforcement Learning Hands-On, Second Edition is an updated and expanded version of the bestselling guide to the very latest reinforcement learning (RL) tools and techniques. It provides you with an introduction to the fundamentals of RL, along with the hands-on ability to code intelligent learning agents to perform a range of practical tasks.
With six new chapters devoted to a variety of up-to-the-minute developments in RL, including discrete optimization (solving the Rubik's Cube), multi-agent methods, Microsoft's TextWorld environment, advanced exploration techniques, and more, you will come away from this book with a deep understanding of the latest innovations in this emerging field.
In addition, you will gain actionable insights into such topic areas as deep Q-networks, policy gradient methods, continuous control problems, and highly scalable, non-gradient methods. You will also discover how to build a real hardware robot trained with RL for less than $100 and solve the Pong environment in just 30 minutes of training using step-by-step code optimization.
In short, Deep Reinforcement Learning Hands-On, Second Edition, is your companion to navigating the exciting complexities of RL as it helps you attain experience and knowledge through real-world examples.
Understand the deep learning context of RL and implement complex deep learning models
Evaluate RL methods including cross-entropy, DQN, actor-critic, TRPO, PPO, DDPG, D4PG, and others
Build a practical hardware robot trained with RL methods for less than $100
Discover Microsoft's TextWorld environment, which is an interactive fiction games platform
Use discrete optimization in RL to solve a Rubik's Cube
Teach your agent to play Connect 4 using AlphaGo Zero
Explore the very latest deep RL research on topics including AI chatbots
Discover advanced exploration techniques, including noisy networks and network distillation techniques
Second edition of the bestselling introduction to deep reinforcement learning, expanded with six new chapters
Learn advanced exploration techniques including noisy networks, pseudo-count, and network distillation methods
Apply RL methods to cheap hardware robotics platforms
目錄大綱
What Is Reinforcement Learning?
OpenAI Gym
Deep Learning with PyTorch
The Cross-Entropy Method
Tabular Learning and the Bellman Equation
Deep Q-Networks
Higher-Level RL libraries
DQN Extensions
Ways to Speed up RL
Stocks Trading Using RL
Policy Gradients – an Alternative
The Actor-Critic Method
Asynchronous Advantage Actor-Critic
Training Chatbots with RL
The TextWorld environment
Web Navigation
Continuous Action Space
RL in Robotics
Trust Regions – PPO, TRPO, ACKTR, and SAC
Black-Box Optimization in RL
Advanced exploration
Beyond Model-Free – Imagination
AlphaGo Zero
RL in Discrete Optimisation
Multi-agent RL
作者介紹
Maxim Lapan is a deep learning enthusiast and independent researcher. His background and 15 years' work expertise as a software developer and a systems architect lies from low-level Linux kernel driver development to performance optimization and design of distributed applications working on thousands of servers. With vast work experiences in big data, machine learning, and large parallel distributed HPC and non-HPC systems, he is able to explain a number of complicated concepts in simple words and vivid examples. His current areas of interest are in practical applications of deep learning, such as deep natural language processing and deep reinforcement learning. Maxim lives in Moscow, Russian Federation, with his family.