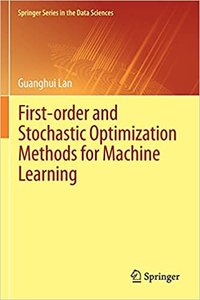
First-Order and Stochastic Optimization Methods for Machine Learning
內容描述
This book covers not only foundational materials but also the most recent progresses made during the past few years on the area of machine learning algorithms. In spite of the intensive research and development in this area, there does not exist a systematic treatment to introduce the fundamental concepts and recent progresses on machine learning algorithms, especially on those based on stochastic optimization methods, randomized algorithms, nonconvex optimization, distributed and online learning, and projection free methods. This book will benefit the broad audience in the area of machine learning, artificial intelligence and mathematical programming community by presenting these recent developments in a tutorial style, starting from the basic building blocks to the most carefully designed and complicated algorithms for machine learning.